Unleashing the Power of Image Datasets for Object Detection
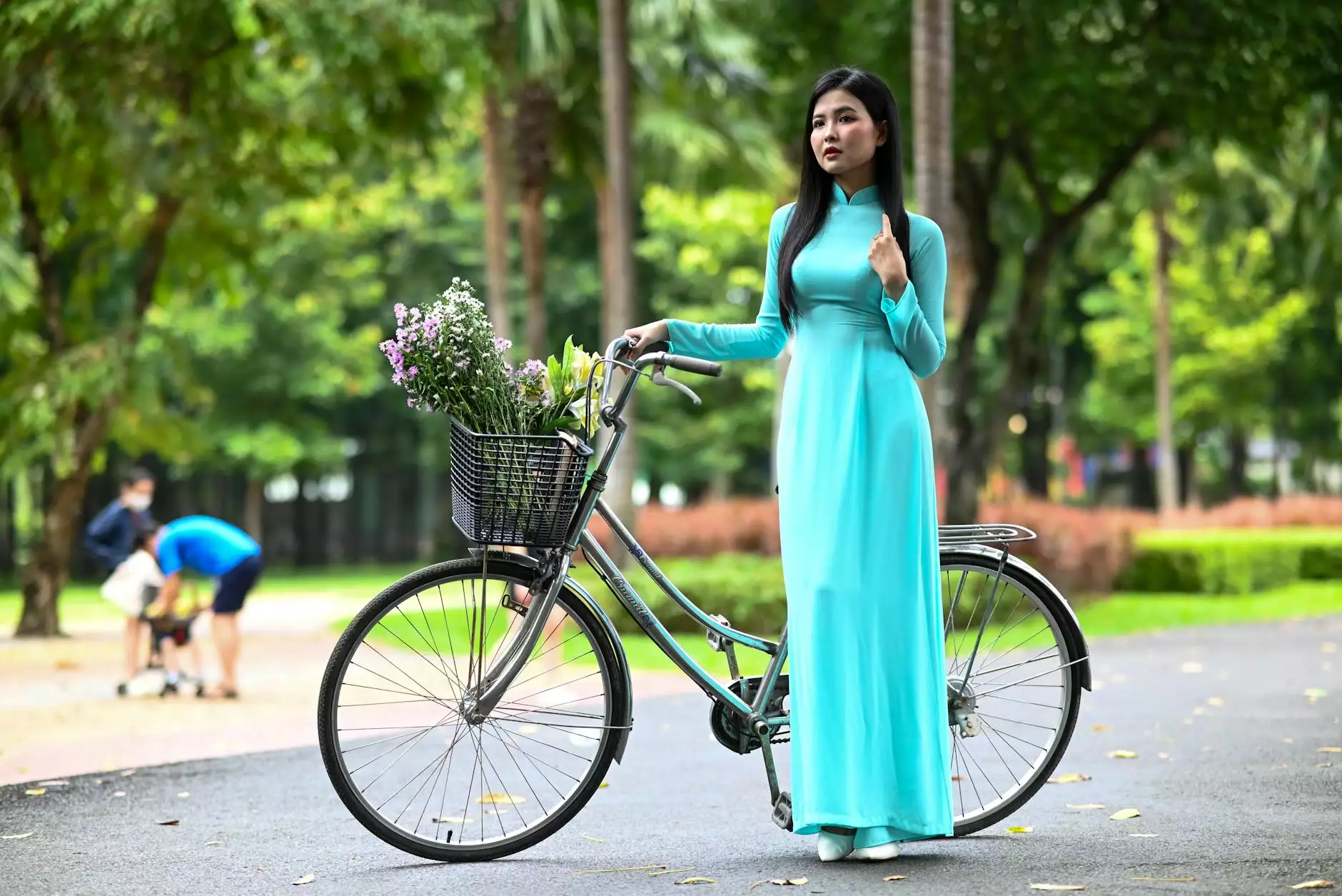
Image datasets for object detection serve as the backbone of machine learning models, empowering them to identify and classify objects within images accurately. In today's fast-paced digital landscape, the significance of these datasets cannot be overstated. Businesses are constantly seeking to leverage advanced technologies, and object detection has emerged as a crucial area of focus.
The Rise of Object Detection Technology
As industries strive for efficiency and innovation, object detection technology has become a game changer. It replaces traditional methods of manually interpreting image data with automated systems capable of real-time analysis. These systems enhance productivity, reduce human error, and unlock new opportunities by enabling a deeper understanding of visual data.
Why Are Image Datasets Crucial for Object Detection?
At the core of robust machine learning models lies a diverse and comprehensive image dataset for object detection. Here are several reasons why these datasets are indispensable:
- Diversity of Data: High-quality datasets encompass a variety of images that represent different angles, lighting conditions, and backgrounds. This diversity ensures that models trained on these datasets can generalize well to real-world scenarios.
- Accuracy and Precision: The precision of object detection models directly correlates with the quality of the training data. Utilizing annotated datasets allows these AI models to learn distinct features and attributes of objects effectively.
- Time Efficiency: Enhanced training times lead to faster deployment of machine learning applications. With well-prepared datasets, developers can streamline their workflows significantly.
Components of a Quality Image Dataset
When building an image dataset for object detection, certain components must be meticulously curated:
- Image Quality: The resolution and clarity of images are paramount. High-resolution images ensure that models can identify even the most nuanced features.
- Annotation Accuracy: Properly annotated data is critical. Annotations must be precise, indicating the exact location of objects within an image.
- Label Diversity: It's essential to include a wide range of object classes in your dataset, allowing models to recognize various items under different conditions.
The Process of Creating Image Datasets
The journey of creating an effective image dataset for object detection starts with careful planning. Here’s a step-by-step guide:
1. Data Collection
Gather images from various sources including:
- Stock photo libraries
- Open-source datasets
- In-house photography
2. Data Annotation
Utilizing data annotation tools facilitates the identification of objects. This process can include:
- Bounding box creation
- Polygon segmentation
- Image tagging
3. Quality Assurance
Conduct thorough quality checks to ensure:
- High accuracy of annotations
- Consistency in labeling across the dataset
The Role of Data Annotation Tools and Platforms
To streamline the creation and management of image datasets, businesses often turn to specialized data annotation tools and platforms. At KeyLabs.ai, we offer comprehensive solutions that simplify the entire annotation process.
Key Features of Our Data Annotation Platform
Our platform provides a suite of features designed to enhance the efficiency and quality of data annotation:
- Intuitive User Interface: A user-friendly design that allows annotators to work efficiently and effectively.
- Collaboration Tools: Teams can work together in real-time, ensuring that all members have access to the latest data and annotations.
- Customizable Workflows: Tailor the annotation process to suit the specific needs of your project, including the option to integrate advanced AI-powered features.
Best Practices for Using Image Datasets
Once you've created your image dataset for object detection, following best practices can greatly enhance the model's performance:
- Regular Updates: Continuously update your dataset with new images to improve model accuracy over time.
- Data Augmentation: Utilize techniques like rotation, flipping, and scaling to artificially expand your dataset size and variability.
- Performance Monitoring: Regularly evaluate the performance of your object detection models and adjust the training data as necessary.
Case Studies: Successful Applications of Object Detection
Here are a few compelling case studies that exemplify the impact of leveraging image datasets in object detection:
1. Autonomous Vehicles
Companies like Tesla and Waymo utilize vast amounts of annotated image data to train their autonomous driving systems, allowing them to recognize pedestrians, vehicles, and traffic signs in real time.
2. Retail Analytics
Retail giants use object detection to monitor customer behavior and analyze foot traffic, leading to more strategic product placements and improved customer experiences.
3. Health Sector Innovations
Medical imaging powered by object detection algorithms is revolutionizing how diseases are diagnosed and monitored, enabling faster and more accurate interpretations of complex images.
The Future of Object Detection with Image Datasets
The evolution of image datasets for object detection continues to unfold, driven by advancements in AI and machine learning technologies. As access to larger datasets and more powerful annotation tools becomes commonplace, we can expect:
- Increased Accuracy: Models will become even more accurate, thanks to better data and algorithms.
- Broader Applications: Industries beyond tech and automotive will adopt object detection for logistics, security, and agriculture.
- Real-time Capabilities: Enhanced processing speeds will lead to real-time data analysis and decision-making in critical applications.
Conclusion
In conclusion, the importance of image datasets for object detection in driving innovation and efficiency across various sectors is monumental. At KeyLabs.ai, we understand that the future lies in harnessing the power of effective data annotation tools and platforms. By investing in high-quality datasets and adopting best practices, businesses not only improve their machine learning models but also position themselves at the forefront of their industries. The road ahead is filled with opportunities, and leveraging the right tools and data can unlock the full potential of object detection technology.