The Role of Machine Learning in Combatting Cyber Threats: Understanding the Machine Learning Virus
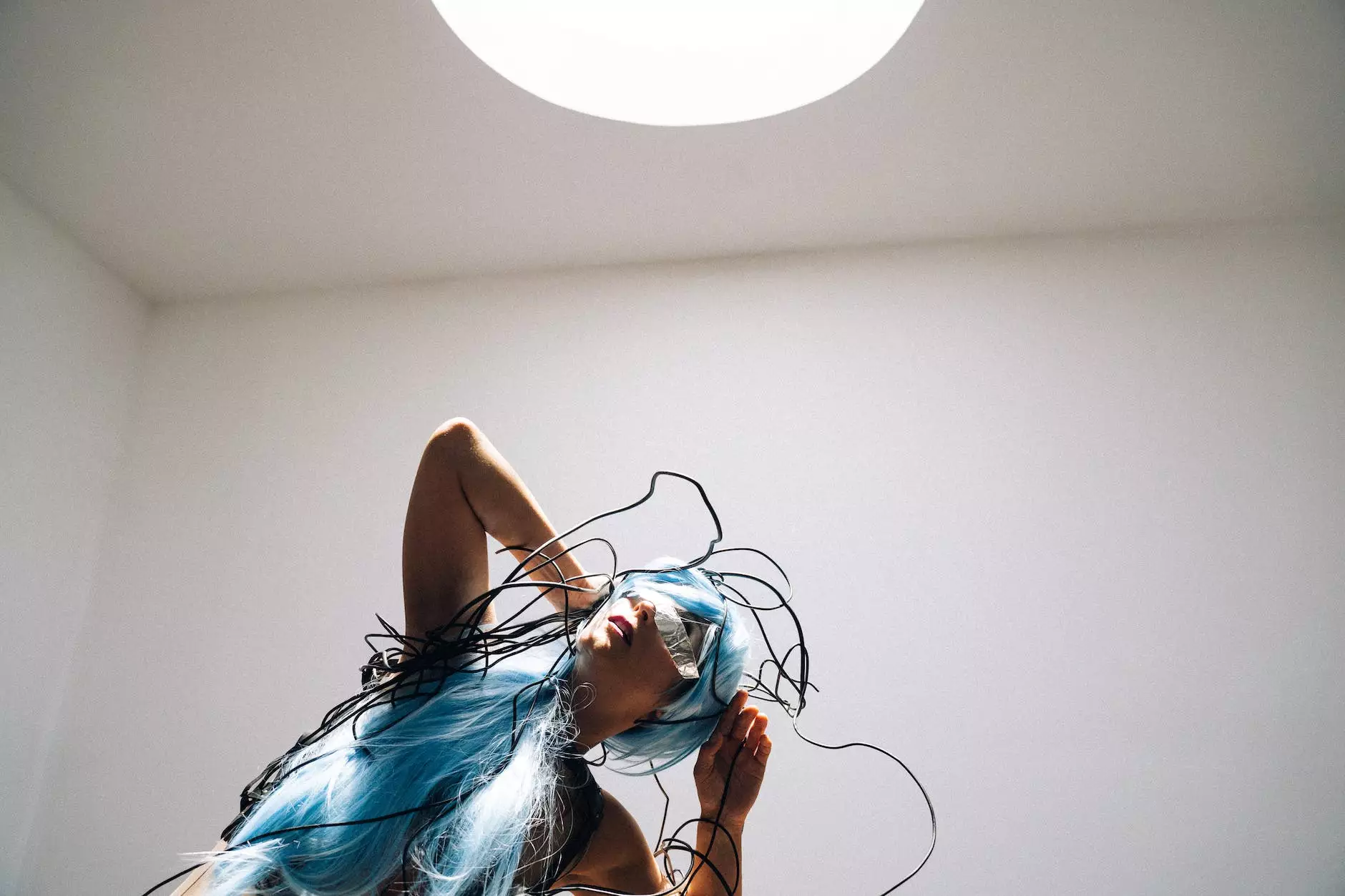
Machine learning is not just a buzzword in the tech landscape today; it is a powerful tool that shapes various industries by enabling systems to learn and adapt from data. In the realm of cybersecurity, the concept of a machine learning virus represents a new frontier where artificial intelligence meets malicious intent. As businesses increasingly rely on IT services and advanced security systems, understanding the implications of machine learning viruses becomes crucial. This article delves into how machine learning is both a guardian and a potential threat in the evolving landscape of cybersecurity.
What is Machine Learning?
Machine learning is a subset of artificial intelligence (AI) that involves the development of algorithms that allow computers to learn from and make predictions based on data. Unlike traditional programming, where explicit instructions are provided, machine learning models improve their performance over time as they are exposed to more data, enhancing their accuracy and efficiency.
How Machine Learning is Utilized in Cybersecurity
Machine learning is reshaping the cybersecurity landscape by enabling organizations to anticipate, identify, and respond to threats in real-time. Among the many applications, the following are key:
- Threat Detection: Machine learning algorithms analyze vast amounts of data from network traffic, user behavior, and system logs to identify anomalies that could indicate a security breach.
- Phishing Prevention: By learning to recognize the patterns typically found in phishing attacks, machine learning systems can flag potential phishing attempts before they reach the end-user.
- Malware Analysis: Machine learning models can classify and analyze malware to understand its behavior and develop countermeasures.
- Incident Response: Automated machine learning systems can facilitate quicker responses to threats, reducing the window for damage significantly.
Understanding the Concept of a Machine Learning Virus
While machine learning is generally perceived as a tool for enhancing security, a machine learning virus is a term that refers to the intersection of AI technology and malware. This phenomenon raises serious concerns regarding cybersecurity:
Defining a Machine Learning Virus
A machine learning virus can be understood as a form of malware that employs machine learning techniques to adapt and evade traditional security measures. Just as legitimate machine learning algorithms improve on their own through data input, malicious versions can learn from the defenses they encounter, refining their strategies to bypass security systems.
Characteristics of a Machine Learning Virus
Understanding the features of a machine learning virus is vital for building effective defenses:
- Adaptive Learning: These viruses can optimize their operations based on the environmental variables they encounter, making them harder to detect.
- Data Poisoning: By introducing misleading data into the training sets of security systems, these viruses can impair the effectiveness of security measures.
- Automated Attacks: A machine learning virus can execute attacks with minimal human intervention, leveraging its ability to learn from past successes.
- Stealth and Evasion: Machine learning algorithms can develop new strategies to conceal their presence, remaining undetected longer than traditional viruses.
The Threat Landscape: Evolving Cybersecurity Challenges
As cyber threats become more sophisticated, organizations must understand the evolving threat landscape driven by machine learning. The introduction of a machine learning virus poses several challenges for IT services and security systems:
1. Increased Complexity of Security Solutions
As malware becomes smarter, traditional security measures may struggle to keep up. Organizations need to invest in more advanced technologies and update their protocols regularly, requiring skilled professionals to manage these systems.
2. The Arms Race of Automation
Security systems and cybercriminals are in a constant arms race—while security technologies become more sophisticated, so do the attacks. The automation of both defensive and offensive strategies creates an environment rife with uncertainty.
3. The Need for Continuous Learning
Organizations must adopt a mindset of continuous learning and adaptation. This involves training machine learning models with up-to-date data to ensure they can respond to the latest threats effectively.
Combating Machine Learning Viruses: Strategies for Businesses
In light of the potential threats posed by machine learning viruses, businesses must adopt comprehensive strategies to safeguard their systems. Here are some effective approaches:
1. Implementing Advanced Security Protocols
Integrate machine learning-based security solutions that can analyze user behavior, detect anomalies, and respond swiftly to threats. Regularly update these systems to adapt to new tactics employed by attackers.
2. Employee Education and Training
Employees are often the first line of defense against cyber threats. Regular training on recognizing phishing attempts and understanding security protocols can significantly reduce risk.
3. Utilizing Threat Intelligence Services
Leverage threat intelligence services to stay informed about emerging threats, including machine learning viruses. Knowledge of tactics, techniques, and procedures (TTPs) used by attackers can enhance preparedness.
4. Adopt a Zero Trust Approach
Implement a Zero Trust security model where no user or device is trusted by default, even if they are within the network perimeter. This approach reduces the risk of infiltration by requiring continuous verification.
The Future of Machine Learning in Cybersecurity
As technology advances, the efficiency and capabilities of machine learning will continue to evolve. In the future, we can expect:
- Enhanced Predictive Analytics: Machine learning will play a critical role in predictive analytics, helping organizations foresee potential threats before they manifest.
- Greater Integration of AI in Security Systems: AI-driven solutions will become more prevalent in security strategies, providing sophisticated tools for threat detection and response.
- Continuous Innovation in Cyber Defense: The ongoing battle against machine learning viruses will drive innovation in cybersecurity tools and methodologies.
Conclusion
The intersection of machine learning and cybersecurity presents both immense opportunities and significant challenges. While machine learning enhances our defenses against cyber threats, understanding the implications and characteristics of a machine learning virus is critical for businesses. By implementing robust strategies, investing in advanced security systems, and fostering a culture of continuous learning and adaptation, organizations can not only protect themselves from these threats but also position themselves as leaders in the rapidly evolving digital landscape.
As we move forward, it is essential for all stakeholders—whether in IT services, computer repair, or security systems—to collaborate and strengthen our collective cybersecurity posture. With proactive measures and an informed approach, we can harness the power of machine learning while mitigating its associated risks.